ChatGPT: The Lab Test Interpreter Extraordinaire – Unveiling Its Superpowers, Gauging Its Safety!
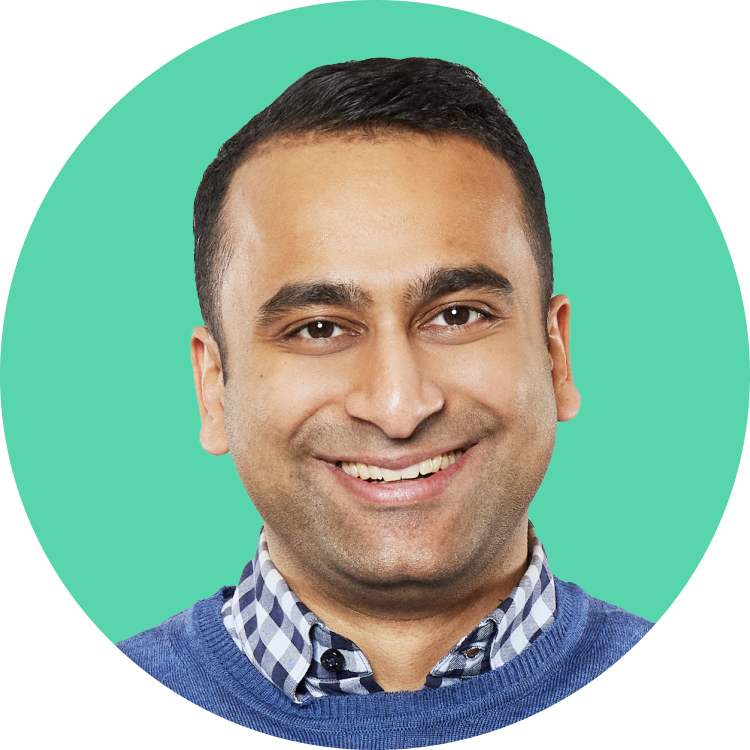
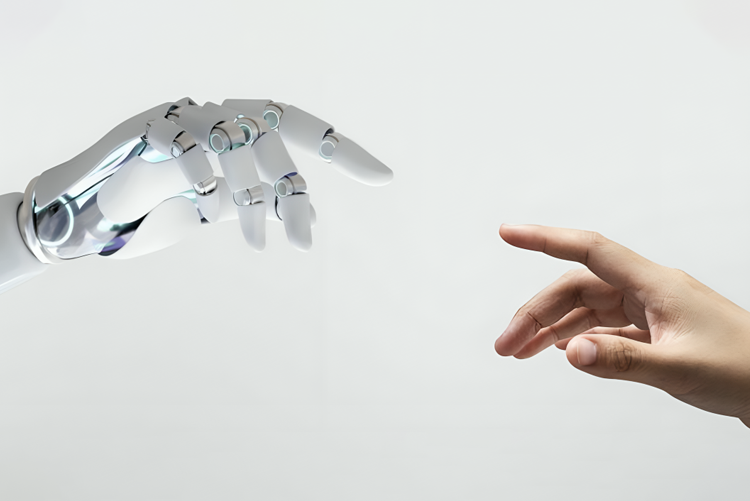
When curiosity strikes, and people find themselves in need of answers, they embark on a quest for knowledge. In the past, the trusty companion on their quest was Google, but now a new contender has stepped into the ring: ChatGPT.
Lab test interpretation is a battlefield where countless individuals seek understanding, and in the European Union alone, over 80 million queries are made each month about the significance of lab test results. While we can’t pinpoint the exact number within ChatGPT’s realm, one can only imagine the sheer magnitude of inquiries. Now, the burning question emerges: Is ChatGPT up to the task? Can it be the heroic solution we’ve been searching for? Let’s find out.
Clinical decision-making systems can be evaluated based on correctness, helpfulness, and safety.
In my quest to evaluate the capabilities of ChatGPT, I discovered that it fancies itself as a savvy anomaly detective in the realm of lab parameters. It confidently explains deviations from reference values, but alas, it falls short when faced with complex cases. For instance, ChatGPT may state that “a low Hct value suggests a lower proportion of red blood cells in the blood volume” or that “low glucose levels may indicate hypoglycemia.” While it generally provides accurate information when interpreting lab test results, it falls short in delivering the practical insights that patients expect.
It seems that ChatGPT’s interpretation skills are stuck in the superficial territory, offering up generic responses like a tired fortune teller. Accurate interpretation requires the ability to juggle multiple result dependencies and recognize elusive patterns, something ChatGPT struggles with at the moment. My extensive testing of ChatGPT’s prowess in lab test interpretation led me down a fascinating rabbit hole, only to stumble upon a research article by Janne Cadamuro et al. confirming my findings. It’s always reassuring to know that even the brightest minds share the same observations.
Now, let’s talk about safety.
Interpreting lab test results without conducting a proper medical interview that involves understanding the patient is not feasible. As an academic teacher at Wroclaw Medical University, I emphasize the importance of thorough medical interviews to my medical students, as they contribute to 80% or more of diagnoses.
Medical interviews go beyond basic information like age, sex, existing diseases, and medications. They should encompass relevant details about symptoms, risk factors, and any evidence linking result deviations to possible diagnoses. Healthcare professionals know that patients often fail to share pertinent medical history, which requires doctors to ask the right questions to reach a differential diagnosis and provide accurate guidance. This is particularly critical in lab test interpretation and can ultimately save lives.
Consider the recent case report of a patient who used LabTest Checker at Diagnostyka, the largest lab test provider in CEE, to interpret his results. This 76-year-old man underwent a comprehensive blood panel, sharing his basic medical history, mentioning arrhythmia and the supplementation of magnesium and potassium. No other complaints were reported. However, during a thorough interview, it was revealed that he was currently experiencing persistent heart palpitations. Given his slightly lowered TSH level (0.329 µIU/ml) and the presence of this symptom, LabTest Checker determined that immediate medical care was needed due to the potential presence of hyperthyroidism leading to life-threatening arrhythmia (atrial fibrillation). Delay of medical assessment could potentially lead to circulatory failure or ischemic stroke, both potentially lethal. Now, imagine if the patient solely relied on ChatGPT’s analysis without undergoing an in-depth interview. It’s not hard to envision a dangerous scenario where he misses a crucial medical intervention because ChatGPT mistakenly indicates that “everything looks fine.”
While ChatGPT generally provides accurate responses, its lack of in-depth interviews can be perilous. Large language models (LLMs) like ChatGPT are susceptible to hallucinations, biases, and generating plausible yet misleading information, making them unreliable for interpreting lab tests.
Although other models like BioGPT and Med-PaLM trained on medical data could offer comparable utility to consulting a physician, their widespread use in healthcare faces significant challenges.
Compliance becomes a major hurdle since these models do not meet data protection laws like GDPR and HIPAA, posing cybersecurity risks. Additionally, the lack of transparency in their development, training, and validation makes effective quality assurance impossible. Relying on these models for medical purposes could be illegal in many jurisdictions and violate professional standards, clinician codes of conduct, medical device regulations, and patient data protection laws. It’s a potential legal minefield waiting to happen.
Moreover, models like ChatGPT are not protected under laws like Section 230 of the 1996 Communications Decency Act since they don’t disclose the sources of third-party information used during training. They are self-contained systems prone to bias and capable of generating plausible yet misleading information. In other words, they excel at being creative rather than providing factual output.
To achieve compliance, ChatGPT would require thorough verification and validation of each component, including the medical knowledge base and the LLM itself. However, this poses significant challenges. Curating and validating a comprehensive medical knowledge base is difficult due to outdated, biased, and location-specific information. The lack of transparency in methodologies used by developers limits access to LLMs like ChatGPT, falling under the category of Software of Unknown Provenance (SOUP), which introduces risks and uncertainties.
More importantly, demonstrating the safety and effectiveness of ChatGPT would require rigorous clinical investigations and compliance with regulatory standards. Conducting a thorough clinical study comparing the model’s performance to that of clinicians would be extensive and comprehensive, following strict criteria and ethical approval. We’re talking about a major undertaking.
From a technical standpoint, achieving all this is not impossible but will require a significant investment of time and expertise. Extensive clinical evidence, literature reviews, feasibility studies, and regulatory-compliant reports would need to be gathered to create a Clinical Evaluation Report (CER). However, obtaining regulatory approval would hinge on the investigation yielding positive results. So, fingers crossed that everything goes according to plan.
nt-weight: 400;”>Let’s put things into perspective by looking back at IBM Watson’s attempt to revolutionize healthcare a decade ago. Despite its groundbreaking technology, top talent, and ample resources, the challenges of implementing AI in healthcare proved to be more formidable and time-consuming than anticipated. Even with the initial surge of deep learning in 2012, it took nearly five years to obtain regulatory approval for an AI-driven device with a very limited scope in breast mammography.
In a nutshell, the emergence of LLMs is set to revolutionize various industries, healthcare being one of them. But hold your horses! We can’t just unleash these models without ensuring their safety. Right now, demonstrating compliance for medical LLMs might seem like trying to fit a square peg into a round hole within existing regulations. However, let’s not lose hope. The future holds the potential for finding a way to make it work and ensure the safe and effective use of these powerful tools. So, stay tuned!